Valid -omics data
Genomics, transcriptomics, proteomics — the list of fields with “-omics” as the suffix has ballooned and so has scientists' excitement and anticipation of what these fields may deliver. When so many biomolecules are tracked at once, scientists can get more detailed and complete pictures of the complex connections between different molecular pathways, cellular and tissue conditions, and pathologies. With the more detailed pictures, researchers can deepen our understanding of biology and even develop novel clinical diagnostic tests or therapeutic treatments to improve public health.
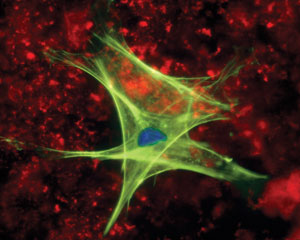
But in the excitement over the promise of -omics technologies, “the issue of validation, an important one, has been a bit neglected,” says James P. Evans at the University of North Carolina at Chapel Hill. He and other researchers, whose expertise range from fundamental research to clinical epidemiology, are worried that if data validation is not properly done, discoveries from -omics endeavors will be pointless.
The notion of validation is not anything new. “The process of replication is a hallmark of science,” says John Ioannidis of Stanford University. Scientists “don’t just blindly trust results, because trust belongs to dogma.”
But experts say that validation of -omics data is a different beast. “For -omics research, the complexity is so immense that we cannot really afford to just go for discovery without validation,” says Ioannidis. “Validation should be built into the process of discovery.”
Hypothesis-generated research -- when one or two variables are tested against one or two others -- tends to produce a few results, which are relatively easy to validate with simple statistical tests. But -omics data sets contain thousands, even millions, of molecules. Because of the sheer quantity of data, Keith Baggerly at the University of Texas MD Anderson Cancer Center says, “I no longer believe that we have good intuition about what makes sense.” Because of this lack of intuition to grasp what large data sets are revealing, Baggerly says these data sets need to be independently verified and checked in multiple ways.
The need for validation is growing increasingly urgent, especially when a significant number of -omics studies are targeted for medical applications. “There is plenty of research that focuses on the initial discovery phase but not enough research on replication, validation and translation,” argues Muin Khoury at the Centers of Disease Control and Prevention, who with Ioannidis recently made some recommendations about the validation of -omics data for clinical studies (1).
Experts all brought up the two cautionary tales of what can go wrong when -omics data are not scrutinized: Correlogic’s OvaCheck test of 2004 and Anil Potti and Joseph Nevins’ clinical trials at Duke University (see Rough patches article). The Institute of Medicine has reviewed how -omics data should be validated for clinical trials (see http://iom.edu/Activities/Research/OmicsBasedTests.aspx).
Much of the emphasis has been on validating -omics data relevant for clinical applications, because patient safety is of utmost importance. But Ruedi Aebersold at the Swiss Federal Institute of Technology in Zurich points out that validation also has significant repercussions in fundamental research. “True, patients aren’t hurt if someone misassigns a protein in a yeast project,” he says. “But it’s still an enormous waste of resources and effort. It’s generally bad for science if the data are poorly reproducible or misassigned.”
Building from the ground up
Like a tower, validation is made of a stack of bricks. The first brick is analytical confirmation. This is the type of validation for which researchers have to ask themselves whether they get the same result from the same experiment done all over again or if a different method on the same sample set gives them the same answer.
Next are the bricks of independent repeatability and replication. Researchers not connected with the original group must see if they can carry out the same experiments and get the same answers. If the analysis has clinical implications, it should also be carried out in larger cohorts to see if the same results emerge.
The next brick of validation is interpretation, and this one “is the toughest of all,” says Ioannidis. “Even when everything has been repeatable, reproducible and replicable, there is some room for differences in opinion.” Ioannidis says that, while he believes in the freedom of researchers to interpret data as they see fit, some standards need to be set in how to interpret data for different fields.
The final brick is asking whether the newly discovered information helps us. “Even if you know what a variant means, and even if it is one you can act on, does acting on it actually improve public health?” asks Adam Felsenfeld at the National Human Genome Research Institute. “It is a huge issue that has to be tackled not just by the clinical community but by health-care economists” and others. He gives the example of the prostate cancer screening test, whose true clinical utility in reducing the burden of disease has been debated. He says that kind of consideration for clinical utility should be built into -omics research as early as possible.
No one-size-fits-all solution
In discussing validation, it’s important to appreciate that the different -omics fields can’t be lumped together. The information gleaned from these fields “encompasses so many different kinds of data. Each one of them has its own technical challenges with respect to validation,” says Ralph Bradshaw at the University of California at San Francisco and co-editor of Molecular & Cellular Proteomics with Alma Burlingame at the same institution. (MCP is published by the American Society for Biochemistry and Molecular Biology.) “If you really want to talk about validation, you have to start piecemeal,” he says, taking each field on its own with its quirks and challenges.
Ioannidis agrees that validation has to be tailored according to the needs of each particular field and the types of measurements available. Just take proteomics. It may have the mission to use large sets of proteins to understand various biological phenomena, but the data come in a variety of forms, ranging from mass spectrometric methods to difference gel electrophoresis. Validation issues for various techniques have to be dealt with in different ways.
As Bradshaw points out, “Validation carries with it the connotation of replication.” He explains that for some -omics fields, such as genomic sequencing, “the replication of the data, both from the terms of technical and biological, is in fact really quite exact.” However, for shotgun proteomics, which identifies by mass spectrometry a large number of proteins from a sample containing millions, “the reproducibility of an experiment, even in the same laboratory on the same sample, is only partial,” says Bradshaw. “You can’t talk about validation [in that case] because of the nature of large-scale mass spectrometry experiments.”
Gilbert Omenn at the University of Michigan, the chairman of the IOM committee on -omics data validation, agrees with Bradshaw. “It’s extremely important to recognize you may not get the same result if you repeat the experiment in the same lab with the same hands with the same samples, because there is a certain stochastic aspect to detection of peptides in mass spectrometry,” he says. But he adds it simply means that there is an even greater need for replication with these types of experiments. While there isn’t a one-size-fits-all procedure for ensuring accuracy of -omics data, Omenn says that no matter the experimental platform, the principles of validation cut across all -omics fields.
Who’s responsible?
Given the magnitude of -omics studies, the responsibility for ensuring that data are valid involves everyone, says Omenn. He doesn’t let anyone off the hook: Students, postdoctoral fellows, principal investigators, departmental heads, institutional review boards, journal editors and funding agencies all have to take their roles seriously to ensure that data are sound.
But in discussing responsibilities, points of contention arise. To validate data, researchers need access to data collected by others. What kinds of data should researchers make available to others? It is important to note, says Robert Chalkley at UCSF, that not every researcher likes the idea of releasing his or her data. It’s not just the risk of scrutiny that alarms these researchers but the worry that someone else may discover something novel in the data that they missed, which can easily happen with -omics research, because the data sets are so large.
But even if researchers see the need for releasing the data, what should they release? It shouldn’t be just raw data, argues Baggerly. He says researchers also should release the algorithms and codes of bioinformatics tools as well as the metadata, the types of information that denote which samples belonged to which groups and how researchers selected those samples. Baggerly explains that, with -omics information, “The data are subject to several different types of pre-processing … In many of these pre-processing steps, any one of several different algorithms could be employed. There is not yet a consensus as to which one is best.” Because there isn’t a consensus, Baggerly argues researchers have to be explicit in stating which ones they used.
Then comes the big question: Who should bear the responsibility of collecting, housing and making accessible all that data? In Baggerly’s view, journals should house the bioinformatics scripts through which researchers ran their data sets for a given publication, because those codes don’t take up much server room. But what about raw -omics data files, which can be gigabytes, even going onto terabytes, in size?
Raw data access
Access to raw data is a thorny subject. One way to illustrate why is to look at proteomics. “Over the years, [raw] data have never left the laboratory in which they were collected,” explains Bradshaw. “It has been clearly the opinion of a lot of people in the proteomics field, and certainly the opinion of the editors of MCP, that these data need to be put somewhere where they can be interrogated by others.”
Websites like PRIDE collect processed proteomics data. But processed data, as Baggerly and Bradshaw are keen to emphasize, are not the same as the raw data spat out by analytical instruments.
So in 2010, MCP made it mandatory for its authors to deposit their raw data files in a repository designed specifically for the purpose. One example of a raw data repository is TRANCHE (https://proteomecommons.org/tranche/), operated by the laboratory of Philip C. Andrews at the University of Michigan.
“For some time, TRANCHE was basically the only show in town,” says Bradshaw. “The problem was that TRANCHE’s funding line eventually was dependent on a [federal] grant, which ultimately was not renewed.”
Over the past year, TRANCHE has struggled, because it hasn’t had funding to hire software engineers who are needed to maintain it. Because of TRANCHE’s technical and financial problems, MCP had to put a moratorium on its requirement for depositing raw data there.
The lack of federal support for publicly accessible repositories for raw data has researchers vexed. TRANCHE isn’t the only example; Omenn, Baggerly and others also point to the Sequence Read Archive, a repository for next-generation sequencing data, which had its funding cut off by the National Center for Biotechnology Information at the National Institutes of Health last year because of budget constraints.
“Funding agencies wish to fund the initial discoveries,” says Evans. For research projects that aim to benefit patients, just producing those first discoveries doesn’t cut it. “You have to spend some time and money ensuring that validation can be done,” he explains. “It isn’t as sexy as funding discovery, but I think funding agencies do have a responsibility to encourage and enable validation. Otherwise, we’re never going to really know which of these discoveries will pan out.”
And unlike funding discovery-driven research, points out Aebersold, it’s not going to cost federal agencies millions of dollars to build and maintain repositories for raw data. Creating infrastructure for data deposition is “not cheap but it’s also not astronomical,” he says. “It’s certainly a serious effort, but it’s not something that would bankrupt the NIH.”
A great example that benefited from public access to data is the Human Genome Project. The organizers of the federally funded project “demanded that data be uploaded, even at a time when the data were riddled with errors,” says Omenn. “It helped clean up the data, because people weren’t hiding it in their own computers!” Because other researchers were able to examine, test and validate the data, genomics has been able to move forward onto whole-genome sequencing, genomewide association studies and other endeavors.
When asked to respond to these views of academic researchers, Lawrence Tabak, a co-chair of the NIH Data and Informatics Task Force and the Advisory Committee to the Director, NIH Data and Informatics Working Group, provided a statement. “Data sharing is critically important to the advancement of biomedical research, and NIH is committed to supporting the collection, storage and sharing of biomedical research data. The astonishing increase in the amount of data being generated through NIH-funded research is an indicator of the extraordinary productivity of the research enterprise,” he said. “Yet with this astonishing increase, the agency is facing significant data management challenges. Given how extremely beneficial the availability of large datasets is to advancing medical discoveries, ensuring its continued availability is a high priority for NIH.”
Tabak, who is also the NIH principal deputy director, went on to say that the NIH director has formed an internal working group as well as a working group to the Advisory Committee to the Director to help inform NIH policy on data management. The committee is expected to make its recommendations in June of this year.
But Bradshaw cautions that having access to the raw data won’t be the entire solution to validation. Raw data access is “not a panacea, but it will make it easier to go in and look at what different people collected under different conditions,” says Bradshaw.
Moving ahead
Experts in this story all cited the volume of -omics data as a cause of concern for validation. But Matthias Mann of the Max Planck Institute of Biochemistry in Germany is hopeful that the data volume issue will someday be more manageable. Right now, the data volume is an indication of the complexity of biology, but some of the complexity of biology comes from interconnections between different molecular pathways, cells, tissues and organs. “I think we will see in the future that many of the biological changes are not independent of each other but they go together,” he says. “That means the dimensionality of what we are measuring is actually lower … That inherently reduces the complexity.” But he cautions, “Until we know more and have mapped it all out, we will be swimming” in data.
The boundaries of biomedical science can’t be pushed forward without proper validation steps, which have to be integrated in all stages from fundamental research to clinical trials and population studies, say Ioannidis and Khoury. Aebersold points out that researchers suffer from lost money, resources and time if they chase mirages in data. And the repercussions of improper validation are magnified if research has medical applications. As Evans puts it, “You get validation wrong, and people will literally suffer.”
References
-
Ioannidis, J.P.A. & Khoury, M.J. Science334, 1230 – 1232 (2011).
-
(2011) NLM Tech. Bull. 378, e15. NCBI To Discontinue Sequence Read Archive and Peptidome. http://www.nlm.nih.gov/pubs/techbull/jf11/jf11_ncbi_reprint_sra.html.
Enjoy reading ASBMB Today?
Become a member to receive the print edition four times a year and the digital edition monthly.
Learn moreGet the latest from ASBMB Today
Enter your email address, and we’ll send you a weekly email with recent articles, interviews and more.
Latest in Science
Science highlights or most popular articles
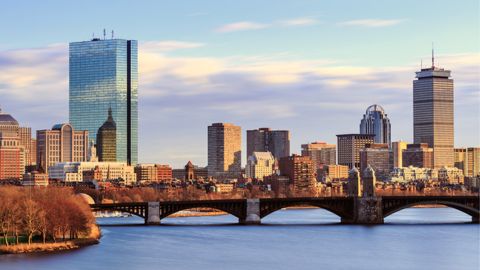
Gaze into the proteomics crystal ball
The 15th International Symposium on Proteomics in the Life Sciences symposium will be held August 17–21 in Cambridge, Massachusetts.
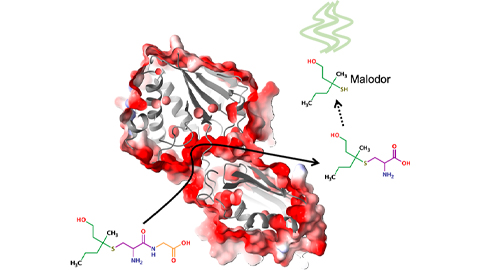
Bacterial enzyme catalyzes body odor compound formation
Researchers identify a skin-resident Staphylococcus hominis dipeptidase involved in creating sulfur-containing secretions. Read more about this recent Journal of Biological Chemistry paper.
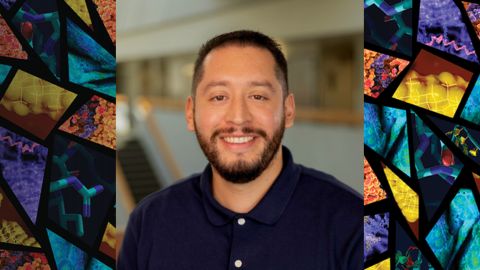
Neurobiology of stress and substance use
MOSAIC scholar and proud Latino, Bryan Cruz of Scripps Research Institute studies the neurochemical origins of PTSD-related alcohol use using a multidisciplinary approach.
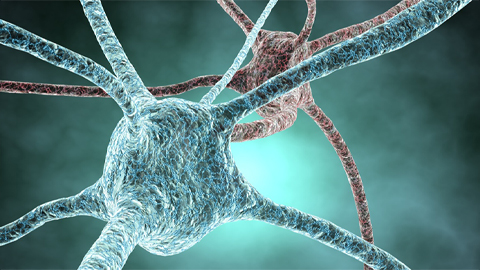
Pesticide disrupts neuronal potentiation
New research reveals how deltamethrin may disrupt brain development by altering the protein cargo of brain-derived extracellular vesicles. Read more about this recent Molecular & Cellular Proteomics article.
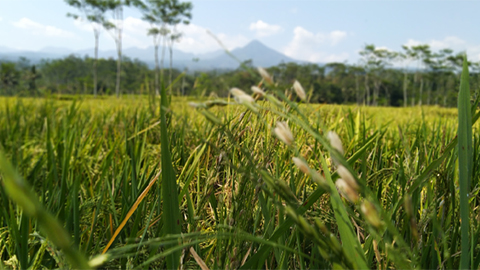
A look into the rice glycoproteome
Researchers mapped posttranslational modifications in Oryza sativa, revealing hundreds of alterations tied to key plant processes. Read more about this recent Molecular & Cellular Proteomics paper.
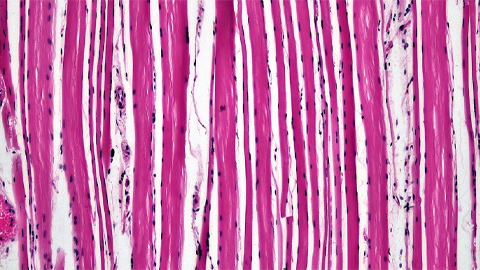
Proteomic variation in heart tissues
By tracking protein changes in stem cell–derived heart cells, researchers from Cedars-Sinai uncovered surprising diversity — including a potential new cell type — that could reshape how we study and treat heart disease.